Unlocking the Power of Data Labeling in Machine Learning
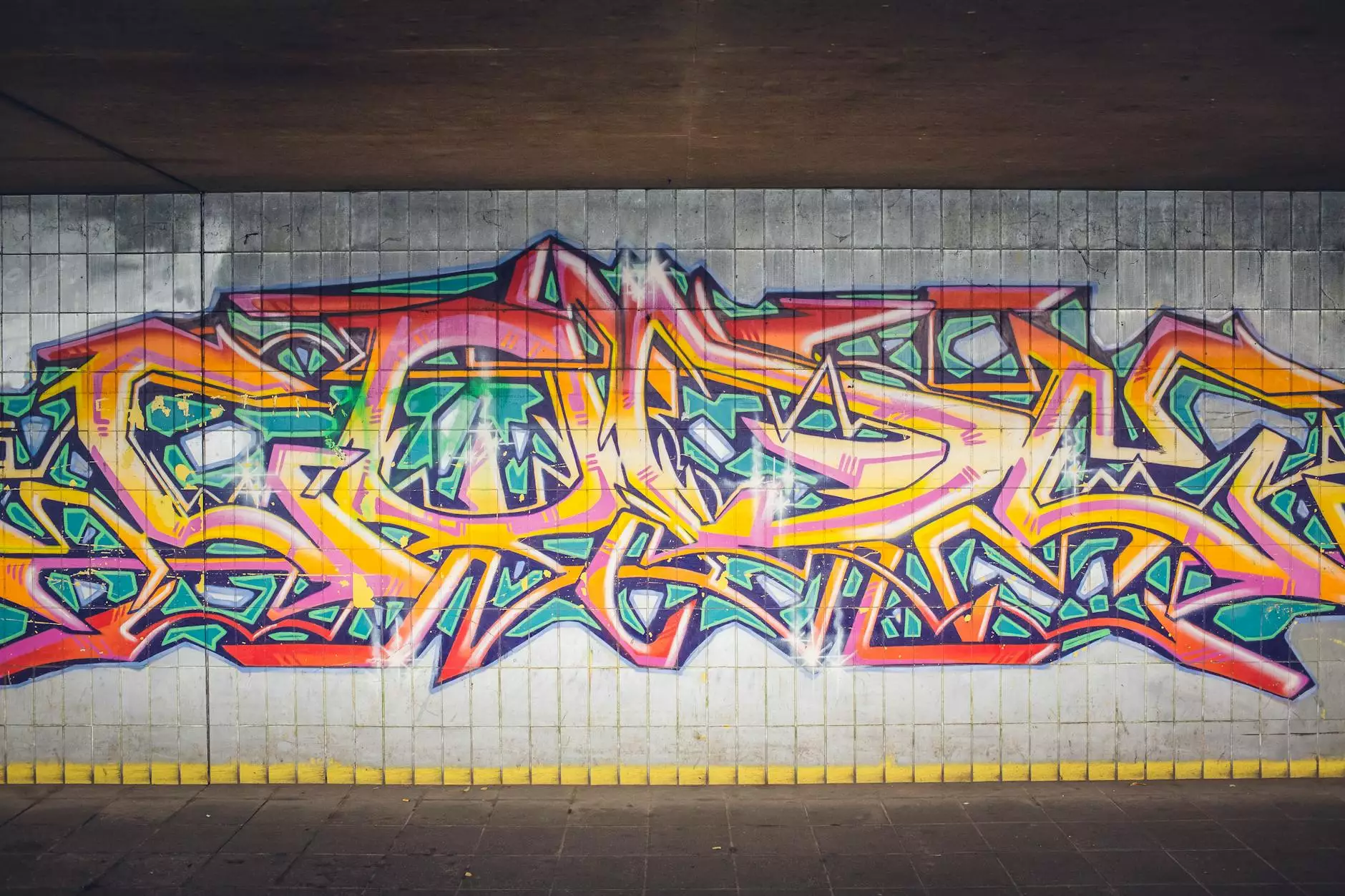
Introduction to Data Labeling in Machine Learning
In the realm of machine learning, the quality of data is crucial to achieving high accuracy and performance. At the heart of this process lies data labeling, a critical step that involves annotating data so that machines can learn from it. As models grow more complex, the demand for effective data labeling strategies continues to rise. Companies such as Keylabs.ai provide cutting-edge data annotation tools and platforms that streamline this essential process.
Why Data Labeling Matters
Data labeling serves as the foundation for supervised learning, allowing models to make predictions based on labeled data. The significance of this process can be summarized in several key points:
- Improved Model Accuracy: Well-labeled data directly influences the accuracy of the machine learning model.
- Enhanced Learning: Labeling helps machines learn from examples, reducing the likelihood of errors.
- Better Insights: With detailed annotations, datasets reveal patterns that can lead to actionable insights.
The Process of Data Labeling
The data labeling process encompasses several stages that ensure data is accurately annotated. Below are the typical steps involved:
- Data Collection: Gather raw data from various sources such as images, text, or audio.
- Labeling Guidelines: Establish clear guidelines that define what each label represents.
- Annotation: Apply labels to the data using specialized tools or platforms.
- Quality Assurance: Implement checks to ensure the accuracy and consistency of the labels.
- Integration: Incorporate the labeled data into the machine learning pipeline.
Challenges in Data Labeling
While data labeling is integral to the machine learning process, it is not without its challenges. Some common hurdles include:
- Time-Consuming: Manual labeling can be a lengthy process, particularly for large datasets.
- Subjectivity: Different annotators may interpret labeling criteria in various ways, leading to inconsistencies.
- Scalability: As datasets grow, scaling the labeling process without sacrificing quality becomes a challenge.
Key Features of Effective Data Annotation Platforms
To overcome the challenges associated with data labeling, an effective data annotation platform should possess several key features:
- User-Friendly Interface: A straightforward interface allows annotators to work efficiently.
- Collaboration Tools: Facilitate teamwork among annotators for better consistency.
- Quality Control Mechanisms: Built-in checks that ensure accurate labeling.
- Scalability: Support for large datasets without degradation in performance.
- Versatile Annotation Options: Options for various types of data including images, videos, text, and audio.
Keylabs.ai: Leading the Way in Data Annotation
One platform that exemplifies these features is Keylabs.ai. With its robust data annotation tools and a user-centric approach, Keylabs.ai streamlines the data labeling process. Here’s how:
Comprehensive Data Annotation Tools
Keylabs.ai offers a wide range of annotation tools that cater to various types of data, ensuring that every project requirement is met:
- Image Annotation: Tools for bounding boxes, segmentation, and landmarking.
- Text Annotation: Options for entity recognition, sentiment analysis, and categorization.
- Audio Annotation: Capability to transcribe and label audio data.
- Video Annotation: Tools to label and track objects across frames.
AI-Powered Workflow Automation
Utilizing advanced algorithms, Keylabs.ai automates parts of the annotation process, significantly reducing the time required for manual labeling. This AI-assisted capability enhances efficiency and allows for greater throughput of data, ultimately improving model training times.
Built-in Quality Assurance
The platform incorporates various quality assurance mechanisms to ensure that the data labeling remains accurate and consistent. This includes peer review options, automated consistency checks, and feedback loops to enhance annotator performance and adherence to guidelines.
Benefits of Using Keylabs.ai for Data Labeling
Choosing Keylabs.ai for your data annotation needs comes with numerous benefits:
- Increased Efficiency: Speedy turnaround times for labeled data allow for quicker model deployment.
- Cost-Effective: Streamlined workflows and automation reduce overall project costs.
- Robust Support: A dedicated team is available to assist users with their data labeling needs.
Real-World Applications of Data Labeling
The implications of effective data labeling extend to various industries and use cases. Here are a few examples:
Healthcare
In the healthcare sector, data labeling is pivotal for training models that can diagnose medical conditions from imaging data. Accurate annotations help improve machine accuracy in identifying anomalies, leading to better patient outcomes.
Autonomous Vehicles
The development of self-driving cars relies heavily on data labeling to annotate images and videos from vehicle cameras. This enables the model to learn how to identify road signs, pedestrians, and other vehicles in real-time.
Natural Language Processing
In NLP applications, data labeling is essential for sentiment analysis, machine translation, and text summarization. Properly labeled datasets allow models to understand context, sentiment, and nuances in language.
Future of Data Labeling in Machine Learning
As machine learning continues to evolve, the future of data labeling looks promising. Here are some trends to keep an eye on:
- Increased Automation: Expect to see more AI-driven solutions that automate data labeling tasks.
- Enhanced Collaboration Tools: Platforms will likely offer better collaborative features for distributed teams.
- Focus on Ethics: Attention to ethical labeling practices will grow as concerns over data fairness and bias become more prominent.
Conclusion
In conclusion, data labeling is a cornerstone of successful machine learning projects. Given its critical role in training accurate models, utilizing a powerful platform like Keylabs.ai can significantly improve the data annotation process. As the landscape of machine learning continues to grow, investing in quality data labeling will undoubtedly pay dividends in terms of performance and insights. By understanding the importance of data labeling and leveraging advanced tools, businesses can stay ahead of the curve and harness the true potential of their data.
data labeling machine learning